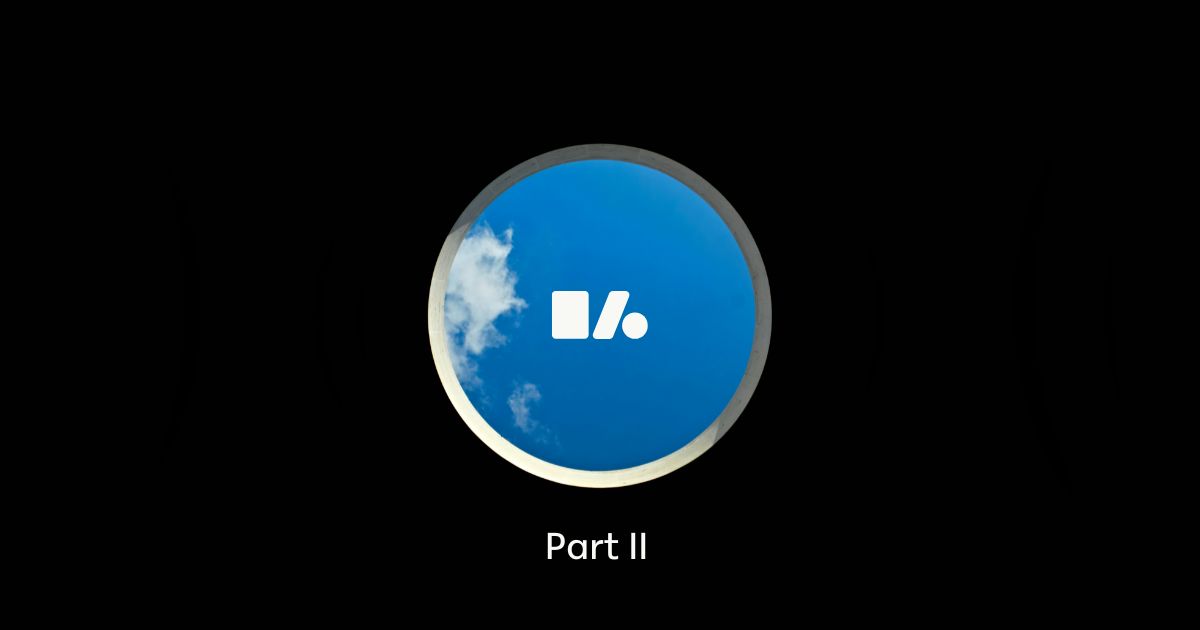
Welcome back to our exploration of the human-AI collaboration landscape. In Part 1, we examined the current state of human-AI teamwork and the complementary strengths of humans and AI. Now, let's dig deeper into real-world collaboration models that are transforming industries today, and what you need to know to implement them successfully in your organization.
Effective collaboration models
Organizations are implementing various models to harness human-AI teamwork.
1. Tiered review systems: Humans monitor and handle exceptions while AI performs tasks autonomously. The human maintains high control in risky situations, making this model ideal for decisions with potentially high stakes. Example: Financial trading algorithms where AI makes trades within parameters, but human risk managers step in when thresholds are exceeded.
2. Human-in-the-loop: Humans act as reviewers/approvers while AI functions as an autonomous worker. This maintains high human control and works best for decisions that always have high stakes. Example: Healthcare imaging where AI analyzes scans but medical professionals review every conclusion.
3. Hybrid/centaur: Humans delegate tasks while AI serves as a specialized assistant. This creates balanced control and works well for complex knowledge work. These teams assign specific subtasks to AI while maintaining human direction and final decision authority.
4. Hybrid/cyborg: Humans collaborate continuously with AI as an integrated partner. Control is fluid, making this approach suitable for creative and analytical tasks. Example: Microsoft's "Copilot" tools where AI suggests edits or code while humans work, creating a dynamic back-and-forth.
Tiered review systems
The AI works autonomously with humans monitoring and intervening when certain thresholds are exceeded or parameters are met. Financial trading algorithms exemplify this approach: AI makes trades within parameters, with human risk managers stepping in only when risk or amount thresholds are exceeded.
Organizations implementing tiered review systems often discover the crucial importance of designing clear, actionable alerts. Initial implementations may flag too many minor deviations, causing "alert fatigue" among human monitors. Refining thresholds based on risk level and creating tiered response systems typically results in more effective and less stressful human oversight¹.
Human-in-the-loop systems
The AI performs a task while humans review outputs. This works well when mistakes are costly and human judgment is crucial (like medical diagnoses or legal reviews).
Healthcare organizations implementing AI for medical imaging can benefit from a human-in-the-loop system. AI systems are reviewing and diagnosing every scan while also categorizing its conclusions based on confidence levels. But whatever the result, a medical professional reviews every conclusion, uses the AI's confidence level as context, and comes to the final decision. This helps increase capacity while maintaining diagnostic quality².
Hybrid collaboration
Humans and AI work together continuously, each contributing in real-time. Microsoft's "Copilot" tools exemplify this approach, with AI suggesting edits or code while humans work, creating a dynamic back-and-forth.
Collaboration patterns
Researchers and industry experts have identified two successful collaboration patterns in human-AI teamwork³:
"Centaurs" (named after the half-human, half-horse creatures of mythology): These teams delegate specific subtasks to AI while maintaining human direction and final decision authority.
"Cyborgs" (representing more integrated human-machine collaboration): These teams integrate AI into their workflow at every step, creating a continuous feedback loop.
Both approaches improved performance, suggesting that effective collaboration might mean allowing individuals to find their personal human-AI balance³.
For any model to succeed, several elements must be in place:
- Clear task allocation: Decide who does what based on comparative strengths
- Effective communication: Design interfaces where AI explains its reasoning and humans can provide feedback
- Well-defined decision rights: Establish who has final say under what conditions
- Continuous improvement: Implement feedback loops where both human and AI performance improve over time
Industry transformation examples
Human-AI collaboration is already reshaping various knowledge work domains:
Professional services
Consulting firms report dramatic productivity improvements when analysts use AI for research and data analysis. JPMorgan's COIN platform for contract review saved 360,000 hours of legal work annually⁴. Law firms increasingly use AI for document analysis, allowing lawyers to focus on negotiation and complex reasoning.
Integrating AI into existing workflows is critical. For example, successful legal document review systems typically embed AI assistance directly within document management platforms that lawyers already use, eliminating duplicate data entry. Effective change management focuses on demonstrating how AI eliminates tedious work rather than replacing professional judgment⁵.
Healthcare
Rather than replacing clinicians, AI extends their capabilities. In medical imaging, AI systems help radiologists by triaging normal scans and highlighting suspicious areas on others, maintaining or improving detection rates while reducing workload⁶.
However, integration challenges in healthcare remain significant. Organizations often find that involving clinicians in the AI development process from day one leads to both better performance and greater acceptance. When physicians help train and refine AI systems, they're more likely to trust and utilize the technology effectively⁷.
Creative industries
Designers use generative AI tools to produce creative concepts, which they then refine and polish. This speeds up iteration while maintaining human creative vision. News organizations and marketing teams employ AI writing assistants for first drafts, with human editors ensuring accuracy and style. The result is often broader exploration of ideas in less time, with humans shifting toward curator and refining roles.
The creative industries provide instructive lessons about human-AI partnership. When implementing AI for content development without clear human creative leadership, the results can be derivative and formulaic. More successful approaches typically involve having human creators direct the AI to explore specific creative directions, which maintains artistic quality while accelerating the development process⁸.
Considerations in human-AI collaboration
As organizations increase their reliance on AI collaboration, several important ethical and legal considerations emerge:
Accountability and decision-making responsibility
One is accountability, or put another way, decision-making responsibility. When humans and AI collaborate, determining accountability becomes complex. Research from MIT suggests that organizations need clear frameworks, including "decision rights", establishing who is responsible for what aspects of collaborative work⁹. This is particularly crucial in high-stakes domains like healthcare and finance where errors can have serious consequences. Documenting both the reasoning behind AI recommendations and audit trails of human interventions can also help.
A second consideration is intellectual property. When content results from human-AI collaboration, who owns the output? The U.S. Copyright Office has ruled that AI-generated content without human creative input is not eligible for copyright protection, but collaborative works remain in a gray area¹⁰. As a starting point, companies can develop clear policies about ownership of AI-assisted work and also document the human creative contribution to collaborative outputs.
A third consideration is privacy and data protection. Organizations must ensure their collaboration models comply with regulations like GDPR and HIPAA. Research from Stanford's Institute for Human-Centered AI highlights that many organizations underestimate the regulatory implications of their AI implementations¹¹. Many of the best practices here are not necessarily specific to AI, for example, having clear data handling protocols and data minimization principles.
Practical implementation guide
For organizations seeking their own human-AI sweet spot, a structured approach is essential:
Step 1: Assess organizational readiness
Many organizations considering implementing AI overestimate their data readiness. Financial institutions and other enterprises often invest heavily in AI infrastructure before discovering their core data is siloed, inconsistently formatted, and rife with quality issues. Starting with a thorough data assessment is essential before significant technology investments.
Here are some dimensions to think about as you evaluate your organization's readiness:
- Data: Do you have accessible, high-quality data for AI to use?
- Technology: Can your systems support and integrate with AI tools?
- Governance: Do you have a clear sense of who in the company should have access to what types of data?
- People: Does your staff have basic AI understanding or willingness to learn?
- Processes: How flexible are your current workflows for innovation?
Frameworks like the MITRE AI Maturity Model¹² can help identify gaps before implementation.
Step 2: Define strategic vision and use cases
Identify specific problems where human-AI collaboration could create value. Prioritize use cases by business impact and feasibility, creating a roadmap with short-term wins and long-term transformations.
Resist the temptation to implement AI for its own sake. The most successful projects address clear business needs rather than showcasing technology. Document expected outcomes with specific metrics to evaluate success.
Step 3: Start with pilot projects
Choose manageable but impactful pilots to develop your collaboration model. Involve users from your employee base from day one, set clear metrics, and contain risk by starting small. Gather success stories and lessons to build momentum.
Organizations finding success with AI implementation often create cross-functional teams that pair technical experts with front-line workers to identify and rapidly prototype solutions. This collaborative approach helps overcome resistance by empowering employees to solve their own pain points with AI assistance, generating excitement about further applications.
Step 4: Invest in training and change management
Typical change management best practices apply here too! Address both technical skills and cultural adaptation. Hold hands-on workshops, identify AI champions, and address fears openly. Recalibrate performance metrics if needed to recognize new collaborative workflows.
Beyond formal training, effective change management often includes practical support like "AI office hours" where experts are available to help colleagues with real-world applications. Seeing AI solve immediate problems tends to create more buy-in than abstract discussions of capabilities.
Step 5: Iterate and scale up
It's unlikely that the very initial implementation of the AI system will be perfect; pilot feedback will help to refine both the AI system and collaboration process. As a system scales, it will have to expand to newer groups of users, including those who may be more resistant to change at first.
Ongoing evaluation
Track both quantitative metrics (time saved, quality improvements) and qualitative factors (employee satisfaction, support tickets). Compare against baseline measurements to demonstrate ROI and identify areas for improvement.
Consider creating a balanced scorecard that captures both efficiency gains and human-centered outcomes like work satisfaction, stress reduction, and time freed for creative or strategic activities.
Avoiding common pitfalls
- Starting without clear objectives: Tie every AI project to specific business goals
- Neglecting data preparation: Invest in data quality before implementation
- Poor change management: Communicate, train, and encourage rather than mandate
- Overestimating AI capabilities: Maintain human oversight and recognize limitations
- Not redesigning workflows: Adapt processes to leverage AI strengths properly
- Scaling too quickly: Expand in stages with checkpoints for adjustment
Each organization's ideal human-AI balance will differ based on business context, workforce capabilities, and strategic goals. The sweet spot emerges through iteration and attentive listening to both your employees and your data.
The path forward
Human-AI collaboration represents a fundamental shift in how knowledge work happens. Rather than fearing replacement, forward-thinking organizations are embracing augmentation, finding the sweet spot where human creativity, judgment, and empathy combine with AI's speed, consistency, and analytical power.
The most successful implementations don't simply automate existing processes, they reimagine how the work is done. When humans are relieved of routine tasks and equipped with AI-driven insights, they can achieve more ambitious and creative outcomes than ever before. The most successful AI + human collaboration models play to the strengths of both humans and AI, elevating the uniquely human elements of work. But getting there requires involving employees when designing the collaboration model.
The future belongs not to organizations that deploy the most advanced AI, but to those that most effectively integrate human and artificial intelligence, finding and continuously refining their own sweet spot of collaboration.
References
¹ Amershi, S., Weld, D., Vorvoreanu, M., Fourney, A., Nushi, B., Collisson, P., Suh, J., Iqbal, S., Bennett, P., Inkpen, K., Teevan, J., Kikin-Gil, R., & Horvitz, E. (2019). "Guidelines for Human-AI Interaction." Proceedings of the 2019 CHI Conference on Human Factors in Computing Systems. https://doi.org/10.1145/3290605.3300233
² Topol, E. J. (2019). "High-performance medicine: the convergence of human and artificial intelligence." Nature medicine, 25(1), 44-56. https://doi.org/10.1038/s41591-018-0300-7
³ Kasparov, G. (2017). "Deep Thinking: Where Machine Intelligence Ends and Human Creativity Begins." PublicAffairs. https://www.publicaffairsbooks.com/titles/garry-kasparov/deep-thinking/9781610397865/
⁴ JPMorgan Chase (2022). "Annual Report 2022: Technology and Innovation Highlights." https://www.jpmorganchase.com/ir/annual-report
⁵ Remus, D., & Levy, F. (2017). "Can Robots Be Lawyers? Computers, Lawyers, and the Practice of Law." Georgetown Journal of Legal Ethics, 30, 501. https://doi.org/10.2139/ssrn.2701092
⁶ Rajpurkar, P., Irvin, J., Ball, R. L., Zhu, K., Yang, B., Mehta, H., Duan, T., Ding, D., Bagul, A., Langlotz, C. P., Shpanskaya, K., Lungren, M. P., & Ng, A. Y. (2018). "Deep learning for chest radiograph diagnosis: A retrospective comparison of the CheXNeXt algorithm to practicing radiologists." PLoS medicine, 15(11). https://doi.org/10.1371/journal.pmed.1002686
⁷ Davenport, T., & Kalakota, R. (2019). "The potential for artificial intelligence in healthcare." Future healthcare journal, 6(2), 94-98. https://doi.org/10.7861/futurehosp.6-2-94
⁸ Frich, J., MacDonald Vermeulen, L., Remy, C., Mackay, W. E., & Biskjaer, M. M. (2019). "Mapping the landscape of creativity support tools in HCI." Proceedings of the 2019 CHI Conference on Human Factors in Computing Systems. https://doi.org/10.1145/3290605.3300619
⁹ Brynjolfsson, E., & Mitchell, T. (2017). "What can machine learning do? Workforce implications." Science, 358(6370), 1530-1534. https://doi.org/10.1126/science.aap8062
¹⁰ U.S. Copyright Office (2023). "Copyright Registration Guidance: Works Containing Material Generated by Artificial Intelligence." Federal Register. https://www.federalregister.gov/documents/2023/03/16/2023-05321/copyright-registration-guidance-works-containing-material-generated-by-artificial-intelligence
¹¹ Stanford Institute for Human-Centered AI (2023). "Artificial Intelligence Index Report 2023." https://aiindex.stanford.edu/report/
¹² MITRE (2023). "AI Maturity Framework and Assessment Model." https://www.mitre.org/insights/publication/mitre-ai-maturity-framework
.png)